Virtual Trainer
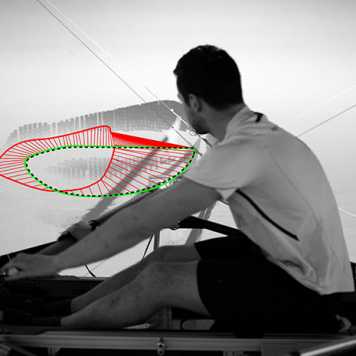
In this project, we develop a virtual trainer to enhance robot-assisted human motor learning. Robot-assisted training enables a lot of different training conditions and settings in task rendering and augmented feedbacks. Additionally, in robot-assisted training, a lot of kinematic or kinetic data is available to evaluate and analyze performance and training efficiency. Within the virtual trainer project we are designing and testing real-time capable concepts to close the gap from automated evaluation of training to reasoned automated selection or configuration of a training condition.
Robot-assisted training is a growing field of application in neuromuscular rehabilition and sports. Thereby, robot-assisted training relieves human trainers or therapists from substantial physical labor. In addition, robot-assisted training can be augmented by virtual reality, providing objective and stimulating feedback as well as motivating game environments. Additionally, kinematic data or interaction forces can be recorded during robot-assisted training to comprehensively monitor performance metrics.
However, selecting and configuring robot-assisted training is challenging. A training session is characterized by the rendered training task (e.g. rowing), the difficulty setting, the robotic support and the chosen feedback. Human trainers are usually not aware of all the possibilities to adjust these characteristics.
However, research in motor learning suggests that selecting robot-assisted exercises carefully is crucial for successful training sessions. Appropriate feedback strategies, the amount of robotic support, skill level dependent task difficulty, and subjects’ motivation have a large impact on the outcome. Therefore, we believe that an appropriate selection and configuration of robot-assisted training has a large impact on training efficiency.
This project applies structured approaches to investigate the effect of robot-assisted trainings on changes in kinematic performance metrics. When robot-assisted trainings and kinematic performance assessments are alternating, the effects of the different trainings on the performance metrics may become observable.
In a first virtual trainer study, we combined autonomous, real-time performance evaluation with the selection of the most effective feedback strategies focusing from previous motor learning studies. Each of these selected feedback strategies focused on special aspects of the rowing task to be learnt. Based on the autonomous real-time performance evaluation, the most promising feedback strategies were selected individually for each rower. In this way, we were the first to realize a human-in-the-loop process for automated human training involving multimodal feedback selection and adaption to the trainee’s needs.
In a second virtual trainer study, we have generalized the concept to a prediction based training selection. The feedback selection was not based on special aspects of the rowing task itself. Instead, the virtual trainer was implemented as a statistically learning agent that directly predicts improvements in spatial and temporal outcome measures dependent on the training configuration and the subjects’ current performance level. From the experiences with this concept validation, we are planning to start transfers of the concept to other fields of robot-assisted training, namely robot-assisted upper arm rehabilitation.
Publications
Georg Rauter, Roland Sigrist, Robert Riener, and Peter Wolf
external pageLearning of temporal and spatial movement aspects: A comparison of four types of haptic control and concurrent visual feedback.call_made
IEEE Transactions on Haptics 8:421-433, 2015.
Roland Sigrist, Georg Rauter, Laura Marchal-Crespo, Robert Riener, and Peter Wolf
external pageSonification and haptic feedback in addition to visual feedback enhances complex motor task learning.call_made
Experimental Brain Research 233(3):909-925, 2014.
Georg Rauter, Roland Sigrist, Claudio Koch, Francesco Crivelli, Mark van Raai, Robert Riener, and Peter Wolf
external pageTransfer of complex skill learning from virtual to real rowing.call_made
PLOS ONE, DOI: 10.1371/journal.pone.0082145, 2013.
Roland Sigrist, Georg Rauter, Robert Riener, and Peter Wolf
external pageTerminal feedback outperforms concurrent visual, auditory, and haptic feedback in learning a complex rowing-type task.call_made
Journal of Motor Behavior 45: 455-472, 2013.
Roland Sigrist, Georg Rauter, Robert Riener, and Peter Wolf
external pageAugmented visual, auditory, haptic, and multimodal feedback in motor learning: a review.call_made
Psychonomic Bulletin & Review 20:21-53, 2013.
Funding
- CRRP Neuro-Rehab: external pagehttp://www.neuro-rehab.uzh.ch/index.htmlcall_made
- Swiss National Funds Grant ”Acceleration of complex motor learning by
skill level-dependent feedbackdesign and automatic selection”, CR23I2 152817.